From Legacy Bottlenecks to 80K/sec Search: How a Global Credit Bureau Scaled with Elasticsearch
The Customer:
A leading global credit bureau operating, offering credit insights and identity verification to financial institutions. Search reliability and performance were critical due to the sensitive nature of PII data.
The Challenge:
The customer’s Elasticsearch clusters were unstable, outdated (v6.1.2), and close to Lucene’s 2B document-per-shard limit. Shared master and coordinating nodes created control plane bottlenecks. Query latency during peak hours and risk of full data loss due to poor shard placement were major concerns.
The Solution:
Ashnik’s Elastic experts re-architected the deployment by separating master/coordinating nodes, enabling Hyper-V–aware shard allocation, and restructuring indices to avoid scale limits. A rolling upgrade path was implemented up to Elastic 8.x. GC tuning and heap optimization significantly boosted search performance—all with zero downtime.
The Benefits:
The customer achieved a 1500% increase in search rate (5K/sec to 80K/sec), 900% boost in business throughput, and reduced search latency from ~300ms to under 2ms. Cluster stability was restored, and long-term scalability was ensured without additional hardware.
Customer Overview
A leading global credit bureau operating delivers credit insights and identity verification services to financial institutions. The organization relies on Elasticsearch to power high-volume, low-latency search queries involving Personally Identifiable Information (PII). Search performance and system reliability are critical to delivering responsive user experiences and timely data access across its operational workflows.
Business Challenge
The organization faced multiple issues with its existing Elasticsearch setup:
- Cluster Instability: If one node went down, the entire Elastic cluster became unavailable.
- Control Plane Bottleneck: Shared master and coordinating nodes created a single point of failure across clusters.
- Scalability Limit: Risk of hitting the 2 billion documents per shard Lucene limit.
- Legacy Versions: The Elastic clusters were running on version 6.1.2, which is end-of-life.
- Performance Issues: Latency during peak query hours and inefficient resource utilization.
- High Latency: Slow response times were impacting downstream applications.
These challenges impacted both system reliability and the ability to meet increasing search workloads.
Challenges Addressed
Node Separation
The customer had three Elasticsearch clusters sharing master and coordinating nodes, which caused performance bottlenecks and created a single point of failure. If the shared master node VMs went down, all three clusters would become unavailable. To mitigate this risk and improve stability, we separated the master and coordinating nodes, ensuring better performance and resilience across clusters.
Cluster Stability
Maintaining high availability was crucial due to the sensitivity and regulatory importance of the data managed. The Elasticsearch cluster was vulnerable to downtime because it operated with a single master node, which increased the risk of split-brain scenarios and instability. To address this, we reconfigured the setup to include three master-eligible nodes, enabling quorum-based elections and significantly improving cluster resilience.
Hyper-V–Aware Shard Allocation
The customer’s virtualized environment involved multiple Elasticsearch VMs deployed per Hyper-V host. Initially, shard allocation didn’t account for underlying physical host placement—leading to primary and replica shards sometimes residing on the same Hyper-V. In failure scenarios, this caused full data loss and cluster unavailability. To solve this, we enabled shard allocation awareness based on Hyper-V topology. Elasticsearch was configured to distribute primary and replica shards across separate Hyper-V hosts, reducing the risk of correlated failures and boosting operational continuity.
Solving the 2 Billion Document Limit
The clusters were nearing Lucene’s 2 billion documents-per-shard ceiling, leading to write bottlenecks and sluggish query performance. Each shard had grown to ~250GB with over 1.6 billion documents—posing a risk of system breakage. We proactively restructured the index to reduce shard sizes to ~25GB and limited each to ~0.25 billion documents. This resulted in a 10X increase in shard count, but it resolved the scale limit for the next 2–3 years and delivered a 2X search performance boost.
Version Upgrade to Avoid EOL Risks
Running an outdated Elastic version (6.1.2) on unsupported OS (CentOS 6) exposed the system to security vulnerabilities and prevented access to newer features. A seamless, zero-downtime upgrade was critical. We implemented a rolling upgrade strategy across all nodes, progressing incrementally through supported upgrade paths—6.1.2 → 6.8.0 → 7.12.0 → 8.x. This allowed us to mitigate compatibility risks while preserving index mappings, configurations, and operational continuity.
GC Tuning for Performance Boost
The Elastic clusters were underperforming due to frequent GC pauses triggered by suboptimal JVM configuration. We assessed the system’s hardware—particularly CPU core availability—and tuned JVM heap sizing and GC flags accordingly. This eliminated long GC pauses, stabilized node performance, and unlocked a 5X improvement in query throughput, all without additional hardware investment.
Architecture Summary
The evolution of the Elastic clusters is a direct outcome of sustained optimization, increased usage demand, and proactive scaling strategies. What began as a lean setup has now transformed into a high-performance, production-grade deployment with significantly enhanced capacity, resilience, and throughput.
- Elastic Cluster 1: 67 nodes (grown from 47), 2TB SSD/node, 144GB RAM, 16B documents, 80,000 queries/sec
- Elastic Cluster 2: 23 nodes (grown from 21), 8B documents, 5000–6000 queries/sec
- Elastic Cluster 3: 8 nodes (grown from 4), 0.23B documents, 1500–2000 queries/se
Each Elasticsearch node was deployed as a VM hosted on dedicated Hyper-V instances to isolate fault zones. To prevent shared control plane dependencies, master and coordinating nodes were also decoupled across clusters to improve fault isolation and resilience. The application layer queried Elasticsearch to retrieve PII-indexed data. Supporting systems like Cassandra and Oracle served as data sources for ingestion pipelines, while Kibana was used for visualization and monitoring.
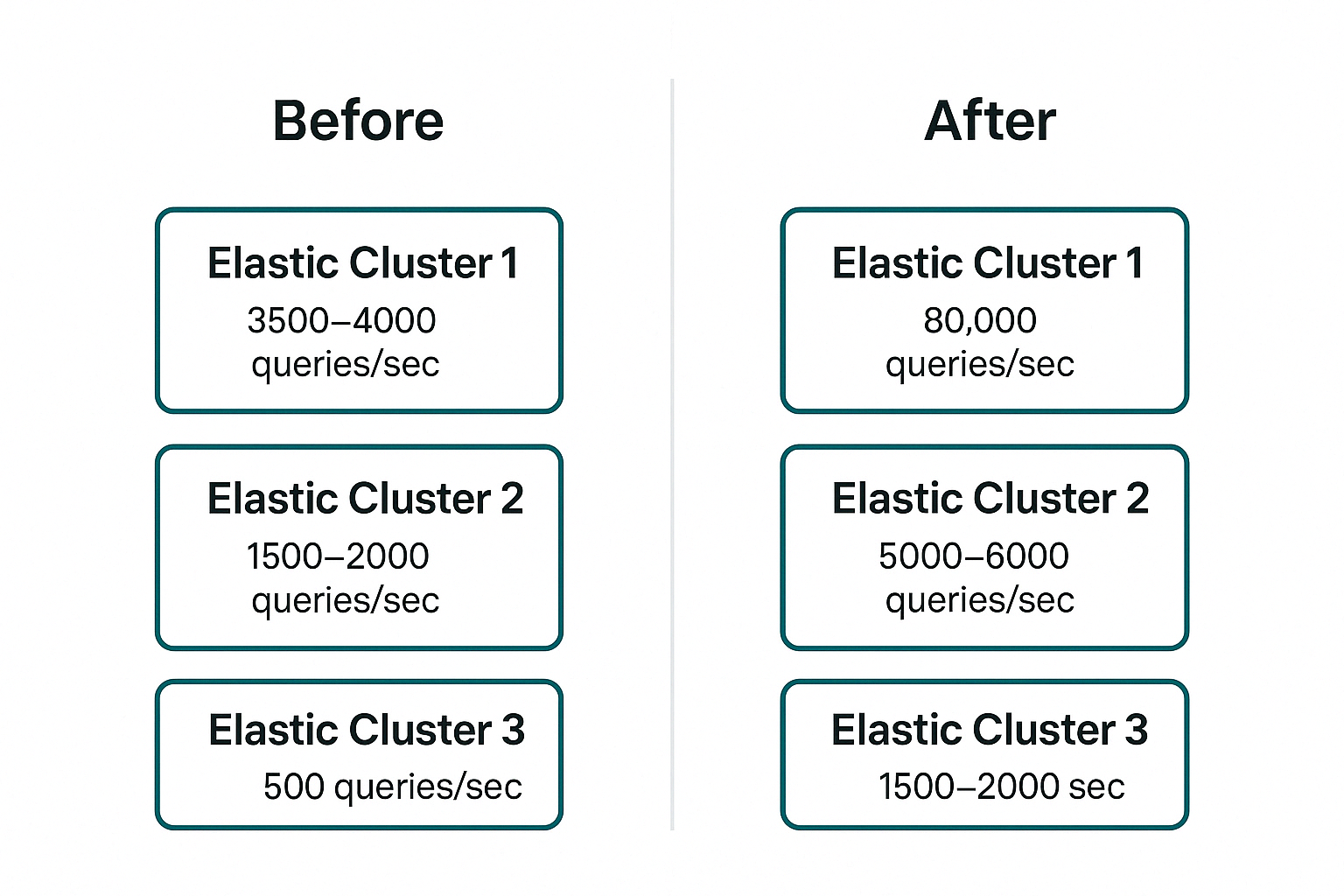
Results
- Achieved a 1500% increase in search rate (from 5,000/sec to 80,000/sec)
- Improved business throughput by 900% (from 1 Lakh to 10 Lakhs)
- Reduced search latency from ~300ms to under 2ms
- Stabilized clusters across Elastic Cluster 1 and Elastic Cluster 3 environments
- Delivered 5X performance improvement without additional hardware investment
- Delivered 5X performance improvement without additional hardware investment
Conclusion
Through strategic upgrades and infrastructure optimization, the organization significantly improved the performance and stability of its Elasticsearch infrastructure. With support during each upgrade cycle and a clear migration path to Elastic 8.x, the customer is now equipped with a resilient, scalable, and high-performance platform.
These outcomes were driven by deliberate architectural decisions, thoughtful execution, and the team’s ability to align performance improvements with long-term platform goals.