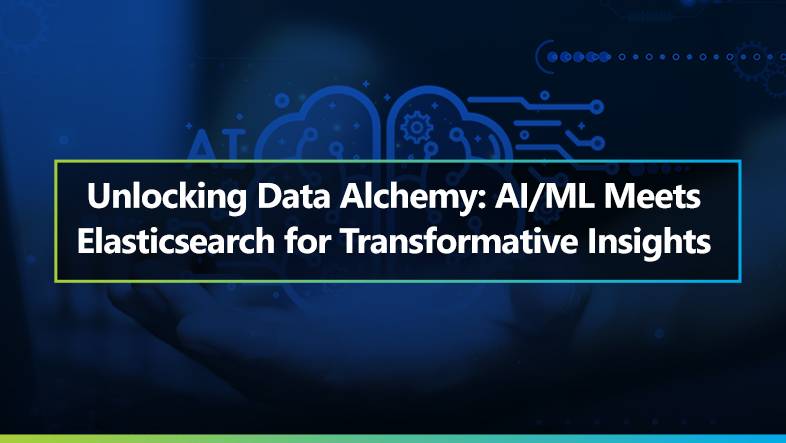
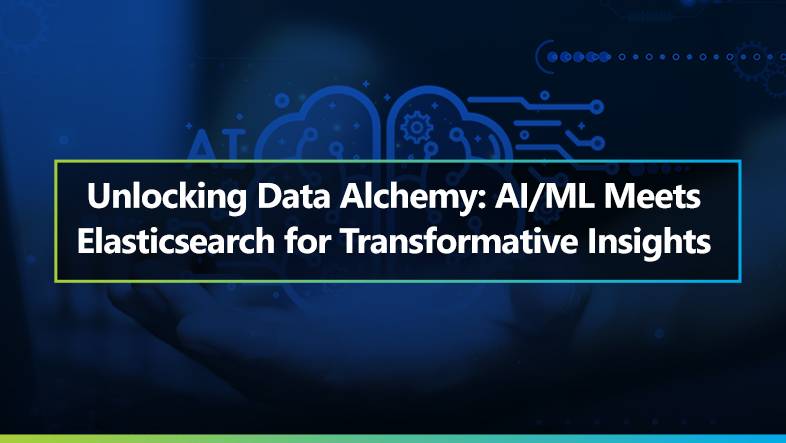
The blog delves into the transformative impact of AI/ML integration with Elasticsearch in data analytics. It emphasizes enhanced data ingestion, NLP-driven search, anomaly detection, personalized recommendations, visual content indexing, real-time search, robust security, and adaptability for evolving ML models.
Table of Contents
Key Highlights
- Diverse Data Ingestion: Elasticsearch’s versatility in assimilating various data types supports the development of complex AI/ML models.
- NLP-Enhanced Search: NLP integration elevates full-text search, enabling advanced sentiment analysis and entity recognition.
- Anomaly Detection and Recommendations: Elasticsearch excels in identifying outliers for predictive maintenance and offers advanced algorithms for personalized recommendations.
- Visual Content Indexing: The synergy with deep learning enables powerful image recognition, expanding capabilities in managing and retrieving visual data.
In the dynamic domain of data analytics, the role of Elasticsearch has been transformative. Its integration with Artificial Intelligence (AI) and Machine Learning (ML) represents not just a trend but a pivotal shift in how we approach strategic decision-making through data. This transformative combination is empowering developers and organizations to refine their data processing, analysis, and retrieval capabilities like never before.
As a developer, I’ve watched the landscape evolve, and I can attest to the fact that the integration of advanced AI/ML techniques with Elasticsearch is indeed a revolution in the field. Here’s a closer look at how this integration is reshaping the analytical capabilities of Elasticsearch.
Firstly, data ingestion has been significantly enhanced. The ability of Elasticsearch to assimilate a wide array of data types and structures is foundational for AI/ML applications. This ensures that data, no matter its form, is quality-rich and readily accessible for the development of sophisticated models. This robust capability forms the bedrock upon which complex AI/ML models are built.
The integration of Natural Language Processing (NLP) has taken Elasticsearch’s full-text search capabilities to new heights. By tapping into leading NLP libraries, Elasticsearch now enables more profound insights, facilitating advanced sentiment analysis and entity recognition. This powerful combination allows developers to extract nuanced meaning from vast tracts of textual data.
Anomaly detection in Elasticsearch benefits greatly from its intrinsic understanding of time-series data. Leveraging the latest in ML models, it has become a prime platform for identifying outliers and patterns that deviate from the norm, essential for predictive maintenance, fraud detection, and more.
Personalized recommendation engines are another area where Elasticsearch shines, thanks to its advanced similarity scoring algorithms. By analyzing user behavior and preferences, ML models, when integrated with Elasticsearch, can deliver highly tailored recommendations, enhancing user experience across various platforms.
The synergy between Elasticsearch and deep learning frameworks has opened up new possibilities in visual content indexing and search. This powerful combination enables sophisticated image recognition capabilities, offering a novel approach to managing and retrieving visual data.
When it comes to model storage, Elasticsearch serves as an efficient document store, streamlining model management, versioning, and deployment. This aspect is crucial for ensuring that the most accurate and up-to-date models are used for inference.
Elasticsearch’s distributed architecture is designed to meet the extensive computational demands of AI/ML workloads. This ensures scalability and high performance, essential for handling the heavy lifting involved in complex data analysis tasks.
The Query DSL (Domain Specific Language) of Elasticsearch now integrates seamlessly with ML models. This allows developers to construct queries that not only fetch data but also apply sophisticated model-driven insights, thus enhancing search relevance and providing richer analysis.
In predictive analytics, Elasticsearch stands out with its real-time search capabilities. As new data is ingested, ML models interfaced with Elasticsearch provide instant predictions, offering foresight and enabling rapid decision-making.
Furthermore, the flexibility of Elasticsearch supports the development of custom plugins, which means that unique ML algorithms can be implemented to cater to specific analytical requirements, pushing the boundaries of what can be achieved with data analysis.
Another key element is data visualization with Kibana. The visual tools provided by Kibana bring data-driven insights generated by ML models in Elasticsearch to life, allowing for a more intuitive understanding of complex patterns.
The open-source community’s role cannot be overstated. Elasticsearch’s compatibility with a plethora of AI/ML libraries underscores its pivotal role in the open-source ecosystem, fostering innovation and seamless integration.
Collaboration within the vibrant communities surrounding Elasticsearch and AI/ML is driving the field forward at an unprecedented pace. The sharing of knowledge and resources accelerates development and ensures that these technologies remain at the cutting edge.
Security protocols in Elasticsearch are robust, a non-negotiable aspect when integrating AI/ML models. Ensuring data and model integrity and confidentiality is paramount, and Elasticsearch’s security features are designed to safeguard these assets.
Lastly, the agility of Elasticsearch in accommodating the iterative and evolving nature of ML models is noteworthy. This adaptability is crucial for models to learn and improve continuously, keeping pace with the ever-changing trends and data patterns.
In conclusion, the convergence of AI/ML with Elasticsearch is a potent combination that is revolutionizing the way we approach data analytics. As we continue to innovate and integrate these technologies, the potential to unlock actionable insights from data is boundless. The journey of integrating Elasticsearch with AI/ML is one that is continuously evolving, and it is an exciting time to be at the forefront of this transformation.
At Ashnik, we recognize the potential of Elasticsearch and design solutions to empower organizations to leverage this cutting-edge technology. To initiate your path towards a more efficient and secure business environment, contact us today.
Let’s redefine the future of your digital transformation journey together