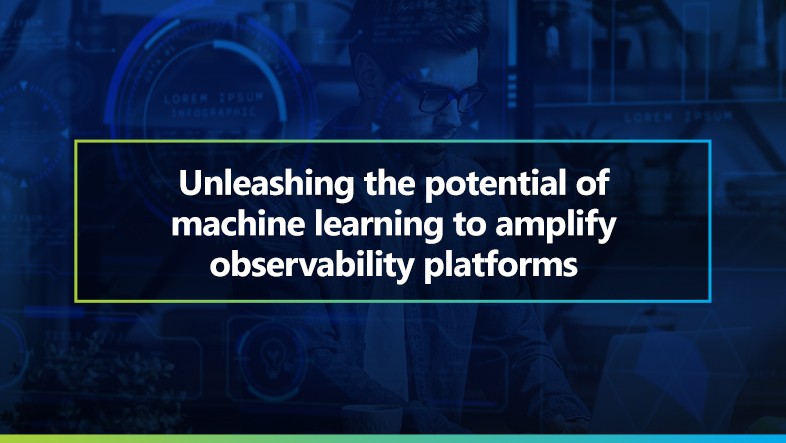
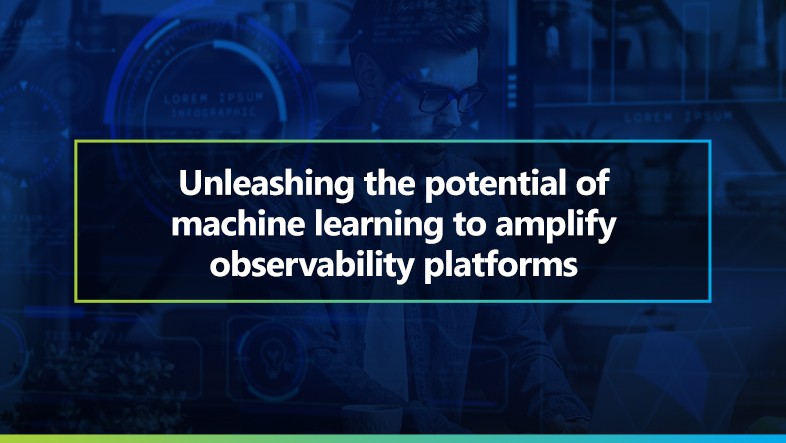
Table of Contents
Introduction
In the age of modern software systems, Observability has become a critical factor in ensuring reliability and performance. As systems grow increasingly complex, the volume and variety of data generated also expands, making it challenging to extract deep insights promptly. Machine learning (ML), when used correctly, can significantly enhance the power of observability platforms, allowing teams to monitor, troubleshoot, and maintain systems more effectively. In this blog post, we will discuss how ML can revolutionize observability and why it is more important than ever to leverage its capabilities.
ML-Driven Anomaly Detection and Alerting
The sheer volume of data generated by modern systems can make it difficult to identify potential issues manually. ML-driven anomaly detection can help overcome this challenge. By training ML models on historical system performance data, we can identify patterns that indicate abnormal system behaviour. These models can then monitor real-time data and detect deviations from the expected patterns, generating alerts when anomalies are detected. This proactive approach enables faster identification and resolution of issues, improving overall system reliability.
Example: An online banking platform experiences sudden spikes in transaction volumes during peak hours, causing slow processing times and delayed transactions. By implementing an ML-driven anomaly detection system, the bank can identify these abnormal spikes in real time and generate alerts for the operations team. This enables the team to quickly investigate and resolve the underlying issue, improving the overall user experience and maintaining trust in the platform.
Enhanced Log Analysis and Clustering
With a growing variety of data sources, log analysis has become increasingly complex. Machine learning can be used to automate log analysis, allowing teams to focus on more critical tasks. By using unsupervised learning techniques like clustering, ML can group similar logs, making it easier to identify patterns and trends. This approach not only speeds up log analysis but also helps in uncovering hidden insights that may otherwise go unnoticed.
Example: An insurance company’s claims processing system generates logs from multiple components, including policy databases, underwriting engines, and customer portals. Manually analyzing these logs can be time-consuming and error-prone. By applying ML-based clustering algorithms, the insurance company can automatically group similar logs together, revealing patterns and trends that help identify potential bottlenecks or failures in the claims processing system.
Importance Of Accelerated Root Cause Analysis
Determining the root cause of an issue is crucial for its timely resolution. ML can assist in this process by analyzing the relationships between different system components and their respective metrics. Using techniques such as correlation analysis and causal inference, ML models can help pinpoint the root cause of an issue, significantly reducing the time spent on troubleshooting.
Example: A mobile payment app experiences intermittent issues affecting users during transactions. Using ML-driven root cause analysis, the app can quickly identify that the problem is caused by a specific third-party API provider. This information enables the operations team to work with the API provider to resolve the issue promptly, minimizing user impact and maintaining the app’s reputation.
Proactive Predictive Maintenance
Machine learning can also be employed for predictive maintenance, allowing teams to anticipate and resolve issues before they escalate. By analyzing historical data, ML models can predict when a particular component will likely fail or system performance may degrade. This information can be used to schedule proactive maintenance, reducing the likelihood of unexpected outages and ensuring optimal system performance.
Example: A data center supporting a hi-tech company’s cloud infrastructure consists of numerous servers, databases, and networking components. By using ML models trained on historical data, the company can predict when certain components are likely to fail or when performance may degrade. This enables the company to schedule maintenance activities proactively, preventing unexpected outages and maintaining a high level of service availability.
Adaptive Monitoring and Dynamic Thresholds
Traditional observability systems often rely on static thresholds to trigger alerts. However, these thresholds may not always accurately reflect the true state of the system. ML can be used to create dynamic thresholds that adapt to changing conditions, minimizing false positives and negatives. Moreover, adaptive monitoring can be employed to allocate resources to different components based on their importance, ensuring that critical components receive the attention that they require.
Example: A cryptocurrency exchange platform collects data from thousands of trading pairs, each generating a unique set of metrics. Static thresholds may not accurately capture the diverse behaviour of these trading pairs, leading to false alarms or missed issues. By employing ML-based dynamic thresholds, the platform can automatically adjust monitoring thresholds based on trading pair behaviour, minimizing false positives and negatives while ensuring critical issues are detected.
Why Machine Learning is More Important Than Ever
As modern software systems become increasingly complex, there is an increasing need for deeper insights and faster analysis. Machine learning is uniquely suited to address these needs, offering several key benefits including:
- Scalability: ML can handle large volumes of data, making it possible to analyze and extract insights from vast and varied data sources.
- Speed: ML algorithms can process data much faster than humans, enabling quicker identification and resolution of issues.
- Automation: ML can automate time-consuming tasks such as log analysis and anomaly detection, allowing teams to focus on high-priority issues.
- Proactivity: ML-driven predictive maintenance and anomaly detection enable teams to identify and resolve issues before they escalate, minimizing downtime and ensuring optimal system performance.
Final Thoughts
In summary, the proper implementation of machine learning can vastly improve the functionality of observability platforms, enabling teams to efficiently monitor, troubleshoot, and maintain their intricate software systems. By embracing observability powered by machine learning, organizations can guarantee the dependability and efficiency of their systems, which can lead to higher user satisfaction for their clients and overall business success for them.
Ashnik is a trusted provider of open-source technology solutions, including guidance on using ML for your observability solutions. Our aim is to provide our clients with reliable, stable, and more efficient support that is aligned with the industry’s needs. With our deep understanding of open-source services, support, and solutions, we are well-equipped to offer expert assistance and help you overcome any challenges with your observability requirements. Trust us to help you with enhancing your machine learning capabilities and maximize its potential.
Get in touch today for a free consultation with our team of experts!